Understanding Kernels in Gaussian Processes Regression#
Using GPy and some interactive visualisations for understanding GPR and applying on a real world data set
toc: true
badges: true
comments: true
author: Nipun Batra
categories: [ML]
Disclaimer#
This blog post is forked from GPSS 2019 Lab 1. This is produced only for educational purposes. All credit goes to the GPSS organisers.
# Support for maths
import numpy as np
# Plotting tools
from matplotlib import pyplot as plt
# we use the following for plotting figures in jupyter
%matplotlib inline
import warnings
warnings.filterwarnings('ignore')
# GPy: Gaussian processes library
import GPy
from IPython.display import display
Covariance functions, aka kernels#
We will define a covariance function, from hereon referred to as a kernel, using GPy
. The most commonly used kernel in machine learning is the Gaussian-form radial basis function (RBF) kernel. It is also commonly referred to as the exponentiated quadratic or squared exponential kernel – all are equivalent.
The definition of the (1-dimensional) RBF kernel has a Gaussian-form, defined as:
It has two parameters, described as the variance, \(\sigma^2\) and the lengthscale \(\mathscr{l}\).
In GPy, we define our kernels using the input dimension as the first argument, in the simplest case input_dim=1
for 1-dimensional regression. We can also explicitly define the parameters, but for now we will use the default values:
# Create a 1-D RBF kernel with default parameters
k = GPy.kern.RBF(lengthscale=0.5, input_dim=1, variance=4)
# Preview the kernel's parameters
k
rbf. | value | constraints | priors |
---|---|---|---|
variance | 4.0 | +ve | |
lengthscale | 0.5 | +ve |
fig, ax = plt.subplots()
from matplotlib.animation import FuncAnimation
from matplotlib import rc
ls = [0.0005, 0.05, 0.25, 0.5, 1., 2., 4.]
X = np.linspace(0.,1.,500)# 500 points evenly spaced over [0,1]
X = X[:,None]
mu = np.zeros((500))
def update(iteration):
ax.cla()
k = GPy.kern.RBF(1)
k.lengthscale = ls[iteration]
# Calculate the new covariance function at k(x,0)
C = k.K(X,X)
Z = np.random.multivariate_normal(mu,C,40)
for i in range(40):
ax.plot(X[:],Z[i,:],color='k',alpha=0.2)
ax.set_title("$\kappa_{rbf}(x,x')$\nLength scale = %s" %k.lengthscale[0]);
ax.set_ylim((-4, 4))
num_iterations = len(ls)
anim = FuncAnimation(fig, update, frames=np.arange(0, num_iterations-1, 1), interval=500)
plt.close()
rc('animation', html='jshtml')
anim
In the animation above, as you increase the length scale, the learnt functions keep getting smoother.#
fig, ax = plt.subplots()
from matplotlib.animation import FuncAnimation
from matplotlib import rc
var = [0.0005, 0.05, 0.25, 0.5, 1., 2., 4., 9.]
X = np.linspace(0.,1.,500)# 500 points evenly spaced over [0,1]
X = X[:,None]
mu = np.zeros((500))
def update(iteration):
ax.cla()
k = GPy.kern.RBF(1)
k.variance = var[iteration]
# Calculate the new covariance function at k(x,0)
C = k.K(X,X)
Z = np.random.multivariate_normal(mu,C,40)
for i in range(40):
ax.plot(X[:],Z[i,:],color='k',alpha=0.2)
ax.set_title("$\kappa_{rbf}(x,x')$\nVariance = %s" %k.variance[0]);
ax.set_ylim((-4, 4))
num_iterations = len(ls)
anim = FuncAnimation(fig, update, frames=np.arange(0, num_iterations-1, 1), interval=500)
plt.close()
rc('animation', html='jshtml')
anim
In the animation above, as you increase the variance, the scale of values increases.#
X1 = np.array([1, 2, 3]).reshape(-1, 1)
y1 = np.array([0, 1, 0]).reshape(-1, 1)
y2 = np.array([0, -1, 0]).reshape(-1, 1)
y3 = np.array([0, 10, 0]).reshape(-1, 1)
y4 = np.array([0, 0.3, 0]).reshape(-1, 1)
k = GPy.kern.RBF(lengthscale=0.5, input_dim=1, variance=4)
m = GPy.models.GPRegression(X1, y1, k)
#m.Gaussian_noise = 0.0
m.optimize()
print(k)
m.plot();
rbf. | value | constraints | priors
variance | 0.262031485550043 | +ve |
lengthscale | 0.24277532672486218 | +ve |
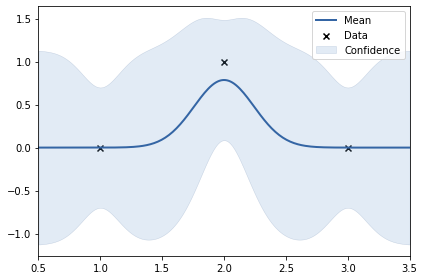
k = GPy.kern.RBF(lengthscale=0.5, input_dim=1, variance=4)
m = GPy.models.GPRegression(X1, y2, k)
#m.Gaussian_noise = 0.0
m.optimize()
print(k)
m.plot();
rbf. | value | constraints | priors
variance | 0.262031485550043 | +ve |
lengthscale | 0.24277532672486218 | +ve |
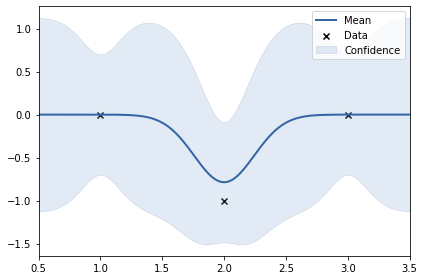
In the above two examples, the y values are: 0, 1, 0 and 0, -1, 0. This shows smoothness. Thus, length scale can be big (0.24)#
k = GPy.kern.RBF(lengthscale=0.5, input_dim=1, variance=4)
m = GPy.models.GPRegression(X1, y3, k)
#m.Gaussian_noise = 0.0
m.optimize()
print(k)
m.plot();
rbf. | value | constraints | priors
variance | 16.918792970578004 | +ve |
lengthscale | 0.07805339389352635 | +ve |
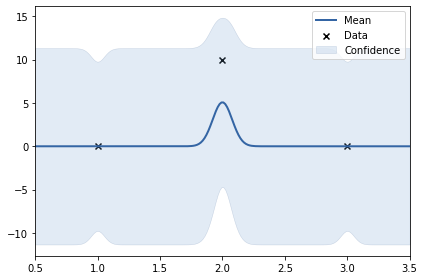
In the above example, the y values are: 0, 10, 0. The data set is not smooth. Thus, length scale learnt uis very small (0.24). Noise variance of RBF kernel also increased to accomodate the 10.#
k = GPy.kern.RBF(lengthscale=0.5, input_dim=1, variance=4)
m = GPy.models.GPRegression(X1, y4, k)
#m.Gaussian_noise = 0.0
m.optimize()
print(k)
m.plot();
rbf. | value | constraints | priors
variance | 5.90821963086592e-06 | +ve |
lengthscale | 2.163452641925496 | +ve |
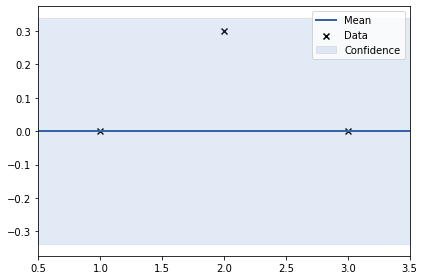