Stationarity of stochastic processes II#
Author: Zeel B Patel
https://bookdown.org/gary_a_napier/time_series_lecture_notes/ChapterThree.html
import numpy as np
import matplotlib.pyplot as plt
from matplotlib import rc
from matplotlib.animation import FuncAnimation
rc('font', size=16)
rc('text', usetex=True)
rc('animation', html='jshtml')
The function below generates multiple samples of \(n\) length, \(d\) order stationary process using the following AR equation (AR stands for auto-regressive),
def AR(phi, n):
order = len(phi)
x = [0 for _ in range(order)]
for i in range(n-order):
tmp = np.sum(np.array(x[-order:])*phi+np.random.normal(0,1))
x.append(tmp)
return x
Let us visualize many samples from AR(\(d=1,n=100,\phi=\{0.8\}\)) stochastic process.
def plot_AR(Phi, n):
X = []
for i in range(201):
X.append(AR(Phi, n))
for sample in X:
plt.plot(sample, alpha=0.2);
means = np.mean(X, axis=0)
std2 = np.std(X, axis=0)*2
plt.plot(means, label='Mean')
plt.fill_between(range(n), means-std2, means+std2, label='Mean $\pm\;2\sigma$');
plt.legend(bbox_to_anchor=(1,1));
plt.xlabel('$t$')
plt.ylabel('$f(t)$')
plot_AR([0.8], 100)
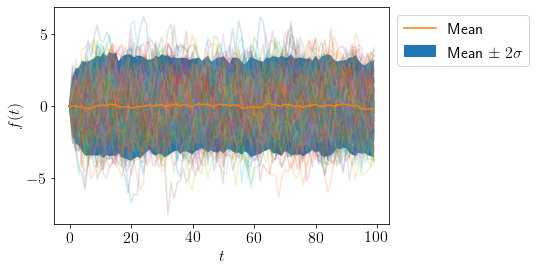
Let us try changing \(\phi=\{-0.8\}\)
plot_AR([-0.8], 100)
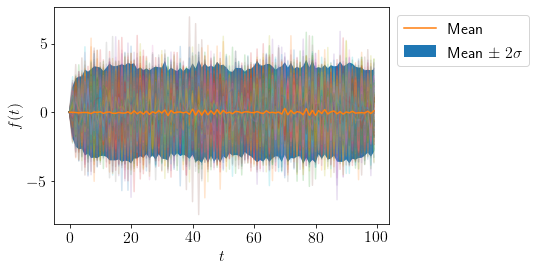
We can see that mean and variance of samples at any time \(t\) is almost constant.
Let us draw some samples from a AR(\(d=1,n=100,\phi=\{1.01\}\)).
plot_AR([1.01], 100)
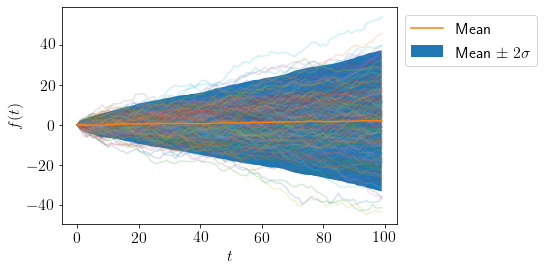
We can see that mean is almost constant over time but variance is varying significantly.
What are the insights? For AR(1) process,
We define charesterstic equation as following,
If roots (magnitude of roots in case of imaginary roots) of \(f_c(B)\) are outside unit circle or in other words, \(|B|>1\), AR(1) process is stationary.
For \(\phi=0.8\), \(B=1.25\) so, AR(\(d=1, \phi=0.8\)) is a stationary process.
For \(\phi=1.01\), \(B=0.99\) so, AR(\(d=1, \phi=1.01\)) is a non-stationary process.
Same rule is applicable for AR(\(d\)) process for any value of \(d\).
Let us try an AR(\(d=3\)) process now.
We take AR(\(d=3,n=100,\phi=\{0.1, 0.2, 0.3\}\)).
plot_AR([0.1, 0.2, 0.3], 100)
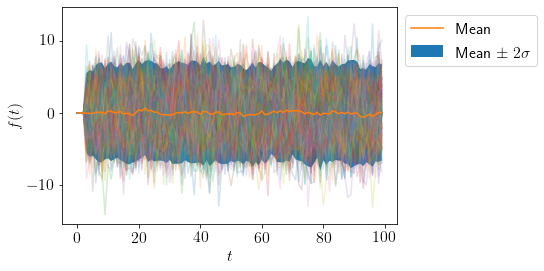
From the graph, process looks stationary, let us find the roots of the following charesterstic equation,
np.roots([-0.3, -0.2, -0.1, 1]), np.abs(np.roots([-0.3, -0.2, -0.1, 1]))
(array([-0.95244656+1.3359895j, -0.95244656-1.3359895j,
1.23822645+0.j ]),
array([1.64073837, 1.64073837, 1.23822645]))
We see that all the roots are outside the unit circle, so the process must be stationary.
Let us try one more example.
AR(\(d=3, n=10, \phi=\{0.6, 0.8, 0.9\}\))
plot_AR([0.6, 0.8, 0.9], 10)
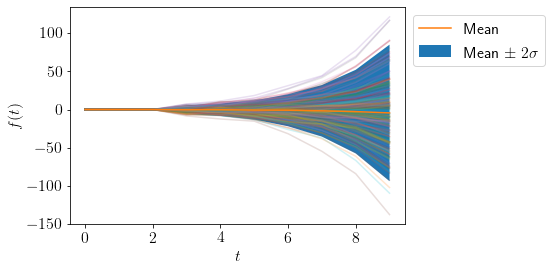
np.roots([-0.9, -0.8, -0.6, 1]), np.abs(np.roots([-0.9, -0.8, -0.6, 1]))
(array([-0.77387424+1.04284911j, -0.77387424-1.04284911j,
0.6588596 +0.j ]),
array([1.29862066, 1.29862066, 0.6588596 ]))
We can see that atleast one root is inside the unit circle, thus the process is non-stationary.